1. Trading systems will use benchmarking to provide intelligence on which algo to use in real-time within the EMS/OMS
2. Increasing emphasis on real-time tools that will interpret TCA results into practical system configurations
3. Core algos will become more intelligent and reactive to market conditions
4. Algos will expand across asset classes - including cross-asset automation
5. Rules will evolve from simple routing instructions to context-based rulebooks measuring market conditions
The algo trading landscape has not evolved significantly for traders over the last few years. Broker algo offerings have been relatively stagnant, and access to specific algos may be limited by the Order Management System (OMS) / Execution Management System (EMS) technology that is in use by the firm.
For clients, algo trading not only provides execution intelligence and automated trading logic, but by passing orders to brokers, traders can make use of liquidity pools that otherwise might not be available to them (e.g., LIS, dark pools, non-member markets, MTFs, and auctions).
The challenge facing most trading desks is that on a day-to-day basis the traders will only use a small subset of available algos and, within the algo itself, a limited selection of available parameters. Using algo trading strategies in production has a minimum required know-how for the trader to select the best algo based on the execution objectives and prevailing market conditions. Without confidence in algo outcomes, traders will often use them as a mechanism for 'handing off' orders to other brokers and directing Best Execution and risk away from their desk.
The market requires a new set of tools to address the complexity of algos reliant on Artificial Intelligence (AI) and Machine Learning (ML). The adoption of ML enables systems to support execution processes by suggesting which Algos to use and the specific parameters best suited for a given objective. Algos will continue to play an important role on the future of trading as market participants strive to find new ways to automate their workflows.
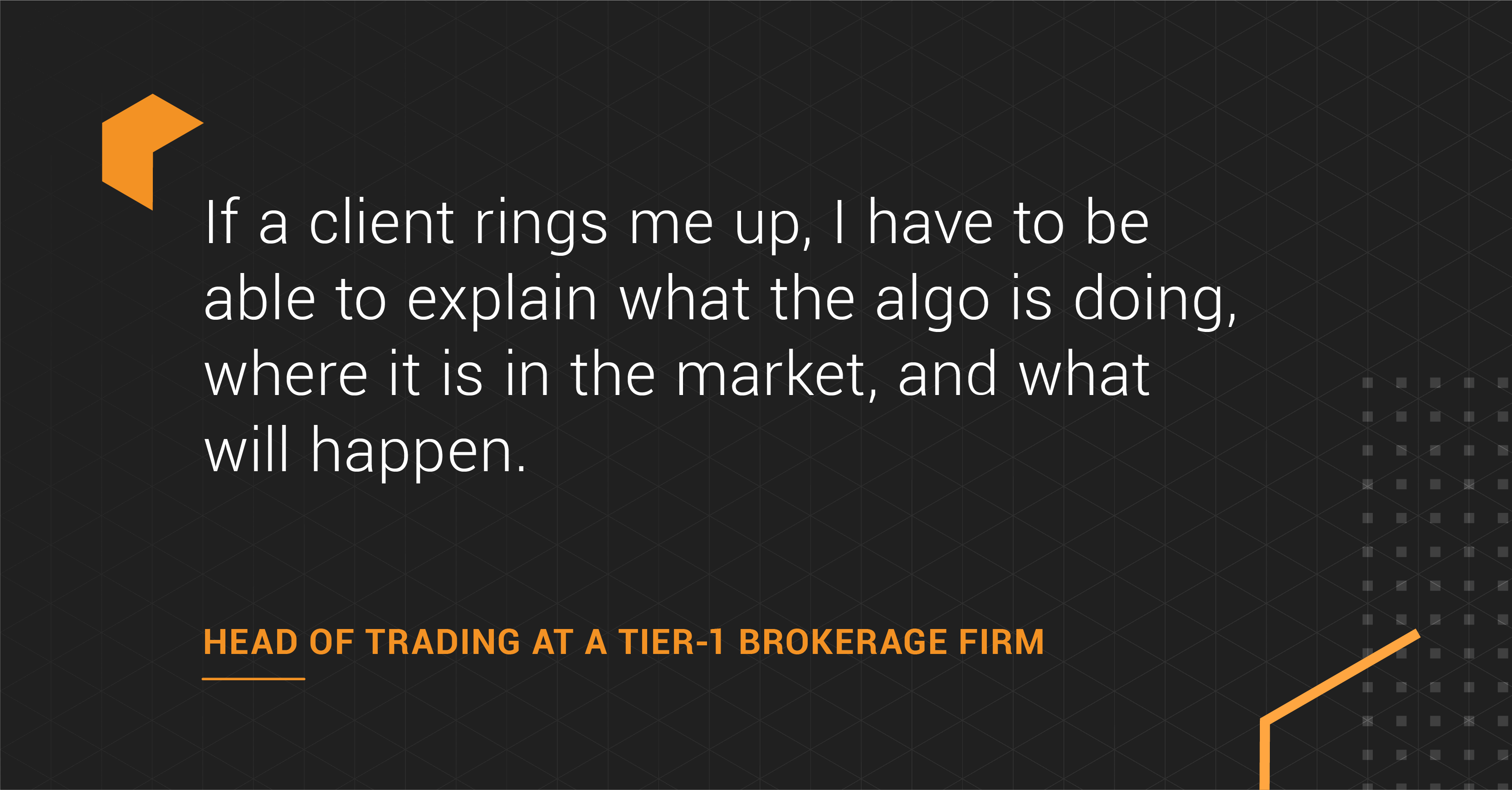
Future of algorithmic trading: trends for 2021 and beyond
1. AI/ML-based selection of dynamic parameters
The problem facing desks today is how to make better use of the available trading strategies, execution processes, and algo capabilities that a trader may be unfamiliar with. Algo trading requires consistent tuning and regular changes in trading infrastructure, which make it very difficult to stay updated.
To date, this responsibility has been with the algo providers themselves, whose sales teams peddle the benefits of specific algos to traders in the hope that the trading desk will choose the right algo at the right time. This methodology has proven to be unscalable and impractical, as one unfavourable result knocks a trader back for months to choosing familiar and easy to explain algo behaviour.
Breaking out of this cycle is the use of AI/ML. In particular, the focus will be on trading systems using benchmarking to provide intelligence on which algo to use in real-time within the OMS/EMS. We see algos generating more intelligent decisions beyond a simple benchmark based algo-wheel, which will help suggest specific settings and parameters using historical data, thereby achieving the best outcomes for the traders.
As algos become very specific to the instrument, market conditions, and available credit present during the trade, more trust will build amongst traders and clients to open a world of opportunities for improving performance and encourage algo providers to invest in their systems. One can envision a world where order management and execution management flow seamlessly utilising automation and machine learning.
2. Real-time integration between algos and Transaction Cost Analysis (TCA)
Since there has been a shift in TCA becoming embedded into both the sell-side and buy-side processes and workflows, traders face similar challenges in figuring methods to use TCA on their desks.
Orders are regularly evaluated for specific performance benchmarks and metrics. However, TCA is only the measurement of an outcome. It does not provide any insight to the trader into how to resolve any poor trading results. As trading technologies and algos have become more complex, it has become near-impossible for a trader to relate poor TCA results to what settings, in a series of complex systems, may influence the trading outcome.
Learn more about how to use ML-driven TCA to improve trading outcomes and decisions in our latest webinar with Refinitiv, an LSEG business.
We will see an increasing emphasis on real-time tools that will interpret TCA results into practical system configurations. That feedback loop will be the essential missing ingredient on every trading desk that makes TCA transformative from a reassuring pat-on-the-back when something goes right into a fundamental tool influencing trading outcomes.
This would include a world where pre-trade market impact models inform broker and algorithm strategy selection, as well as route eligible order based on pre-defined rulesets, helping traders intelligently decide the most appropriate execution algo for the required objective and on which venue.
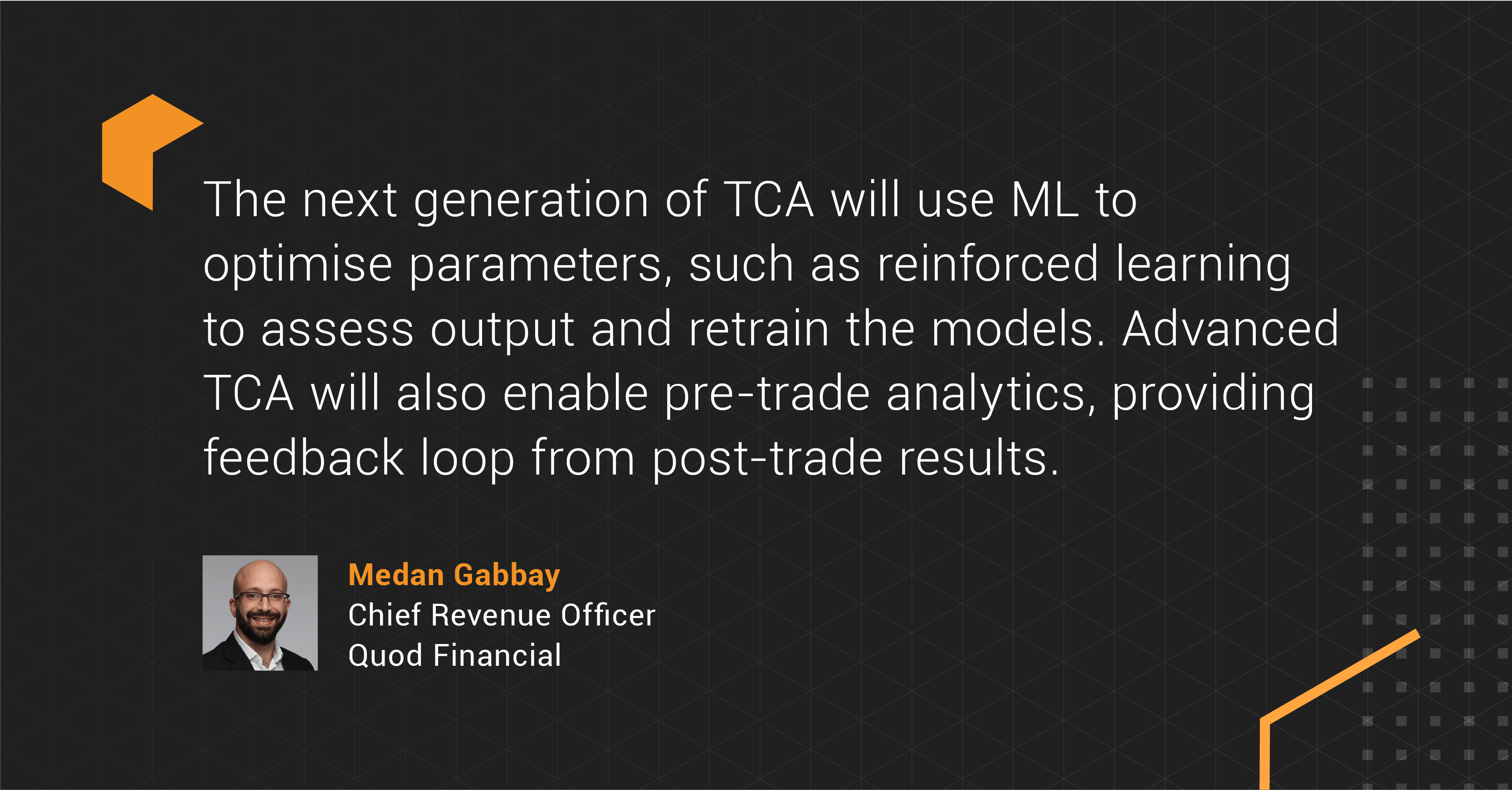
3. Pre-trade recommendations for better insight into performance
As the menu of algos and the range of configurable settings available to traders continue to increase, traders are suffering from information overload and simply sticking to their favourite top 4 - 5 algos.
Asset managers, however, have seemingly become less willing to send larger volumes and are opting more and more towards smaller notional quantities using Implementation Shortfall (IS) or Auctions. Indeed, in current trading, market participants have opted for algos to split up large orders into smaller digestible pieces that can be dispersed across different venues and executed against multiple liquidity pools to improve the quality of their executions and to help their clients reduce trading costs.
With that, we will see core algos becoming more intelligence and reactive to market conditions, for example, with the introduction of Quod Financial's AI/ML-powered Peg-Offset behaviour (product available in late 2021).
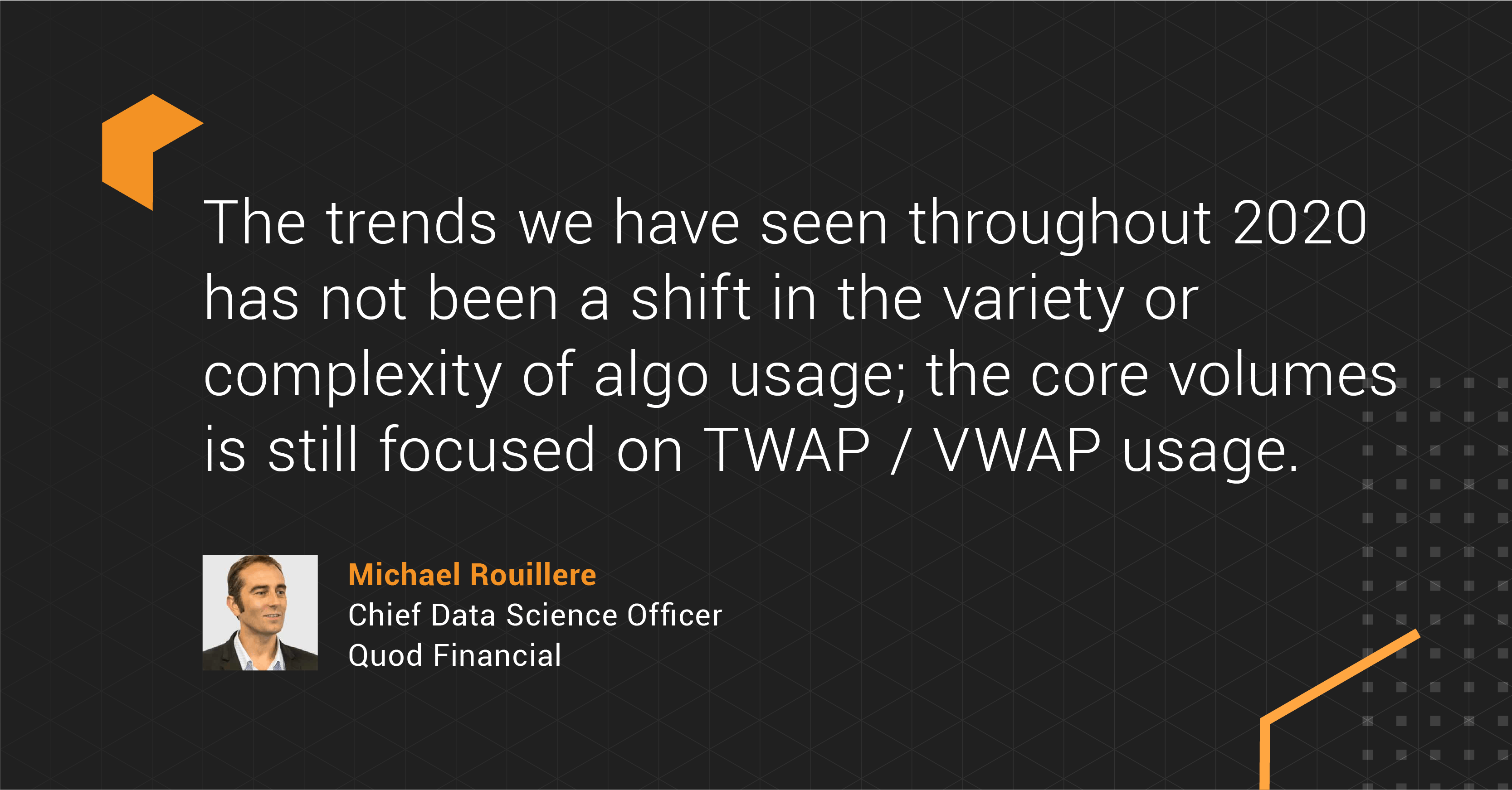
In this scenario, the child slice of any other algo type can be a peg that uses a dynamic offset from a given benchmark driven by AI/ML predictions of micro-volatility and market events. The peg itself naturally increases the effectiveness of passive trading within any algo by combining it with predictive mechanisms to anticipate small market changes that will allow traders to capture a few additional basis points (BP) on all use cases. This also helps brokers introduce new features of algo trading without going through the challenging process of convincing end clients to trust more exotic algo trading behaviour.
Moving ahead, as pre-trade recommendations give better insight into the performance of all available algos, we expect traders to make use of a wider range of strategies that are appropriate for a given stock and market condition. For traders, this feedback loop of AI/ML-driven recommendations helps provide more pre-trade confidence of which broker algo or customised algo to use in any given situation.
The third generation of algos will be adaptive and will deploy ML-driven technology and real-time insights so that algos can self-adjust when working the trade.
4. Algos to take more prominence across multiple asset-classes
Another significant trend that has taken prominence in 2021/2021, and we see gaining more momentum in the future, is the expanded use of algos across asset classes - including cross-asset automation. This is also an extension to the trend of moving away from basis point charges to Tier-1 brokers and leveraging the fact that multi-asset trading technology can make these trading strategies available to all desks at no additional cost. Examples include simple automation like Smart Order Routing (SOR) on FX Forwards and full algo trading strategies on derivatives.
In an article written by The Trade, Hayley McDowell and Peter Ward, Global Head of Futures and Options Electronic Execution at JP Morgan, support this trend and discuss the growth of fixed income (FI) futures algorithm. The Trade reveals that "since 2016, futures volumes traded via algos at JP Morgan have increased 40% year-on-year. Algos now comprise almost 20% of the bank's total futures trading flow, up significantly from roughly 4-5% in 2016 and 2017."
In another case, JP Morgan records that in 2016, clients traded <35% of their eFX Spot volume via algo orders. In 2020, this increased to almost 60% - displaying a watershed year for FX algo execution. It is going to be difficult to ignore the advantages of FX algos. In FX trading, algos allow institutions to make better execution decisions, minimise trading costs, capture time stamps on each fill, and stealthily place trades across multiple venues.
5. Algo usage for more automation and efficiency
Finally, the growth we have seen extends into automation. As trading desks are pressured to handle client orders faster, with fewer traders and increasing volumes, the only solution is automation. Rules have evolved from simple routing instructions to context-based rulebooks measuring market conditions. For example, volatility, depth, hit ratios, latencies, and real-time client information such as P&L over time, recent orders, and current order books.
Building these complex rules allow total automation of flow and to make recommendations of particular routes and strategies. That way, traders can speed up their decision making at the point of trade. This can include cross-asset behaviour such as auto-generation of FX legs for non-listed currency-denominated orders.
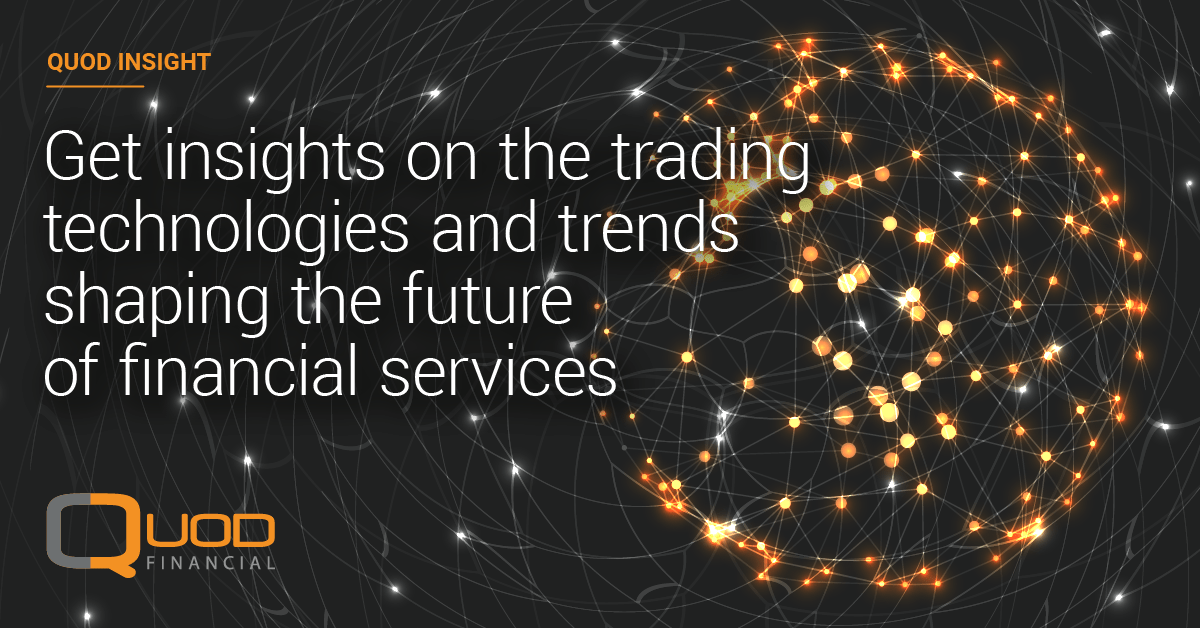