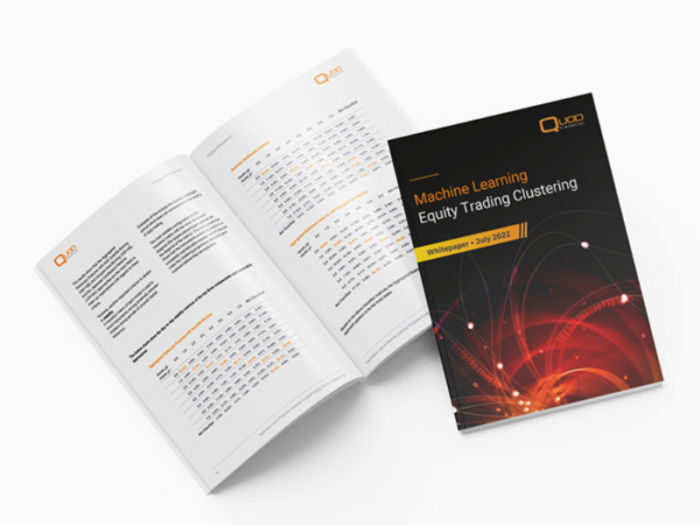
- v1.0 Version
- 761 Download
- 800KB File Size
- 1 File Count
- 2nd August 2022 Create Date
- 4th August 2022 Last Updated

The equities universe, from shares of companies to ETFs and Warrants, is greater than 4 million instruments. Some are liquid, some not particularly liquid, and some are traded sporadically. The prevalence of automated electronic trading, combined with the use of algos and smart order routing, results in an increasing use of historical data. This is present in an array of trading, risk, and execution decisions for example VWAP Algorithms or Percentage of Volume (POV) or Implementation Shortfall (IS) algos.
The first addressable issue relates to the sheer volume of data that needs to be captured, cleansed, stored, and processed for execution systems to use in real-time. Often, the data for these decisions is insufficient, as a result of licensing, granularity or simply the
data is unavailable.
This whitepaper aims to explain a fully data driven approach to identify groups of instruments that perform similarly over short periods of time using Machine Learning cluster analysis. This method is used to reduce the instrument universe required to perform data-driven operations.
File |
---|
Machine-Learning-and-Clustering-Whitepaper-July-2022.pdf |