Capital markets have traditionally been a leader in the adoption of new technology, and Machine Learning (ML) is no exception to this trend. The full electronification of trading is now being revolutionised by AI and ML.
- The 2020 Algorithmic Trading Market report stated 60-73% of US equity market trades utilise algorithmic trading
- Refinitiv stated that foreign exchange (FX) algo trading, as a percentage of FX spot volumes, has now risen to 20%
- A 2019 survey by JP Morgan estimated 80% of their FX flow would come via electronic channels.
"To start any AI journey, you must first overcome the challenge of data. Data starts and ends each and every AI/ML project"
The connectivity and big data challenge in trading and financial services
There’s no denying that data’s an incredibly valuable resource. But, there are some data themes that are getting overlooked in the industry due to a number of challenges.
One of the biggest ones in financial markets today is data availability. Clients often find that key data isn’t always readily available, or it’s too costly to produce in real-time. Sometimes, physical location, or hardware requirements to process data, can also make it prohibitively expensive. In some asset classes, such as OTC markets, data may be hard to obtain or license.
If an organisation manages to overcome availability, they may face issues with data quality and consistency. As the volume of data grows, it can quickly become obsolete. If firms cannot process information quickly, they may produce invalid or misleading conclusions. Data must be consistent and relevant for traders to understand market trends or to perform market analysis. Poor quality data could result in traders making loss-making decisions or re-executions.
In terms of consistency, common issues such as accurate timestamping and clock synchronisation have been resolved under MiFID II. But, there are still significant issues with how data is represented and reported due to inconsistencies between providers and sources. This creates significant challenges for data consistency in the industry.
Data privacy is also a significant challenge for clients and market participants. In OTC markets, data is frequently offered on a counterparty basis to different tiers or individual clients. The price is based on a combination of factors. This could include derivation of credit / rating, volume traded, relationship and nature of the flow. In listed instruments, for instance, the availability of real-time data or access to markets, as well as rights of redistribution, creates groups of people with very different information. This data can be both expensive and often impossible to obtain.
Adding to the challenge tally is agreeing benchmarks. In a fragmented market, much of the liquidity is hidden from market participants. Within the OTC framework, it’s impossible to tell if the prices are in line with the wider market. Understanding a true market price is a challenge for all parties – the many fixing scandals and market tape initiatives are testament to this.
As time passes and data is consumed, data accumulation creates an issue for storage and transport. Physically locating data in trading systems is expensive, but low-cost storage can create challenges with data transfer performance. Large data volumes also increase processing costs. As data grows, the implementation of a scalable solution is a key requirement – altering the techniques to process increasingly complex datasets.
As vendors realise the value of data and the growing pressure to reduce costs, they increasingly attempt to commercialise alternative data in environments where they are being pressured to reduce other prices. Obtaining data from multiple sources can exponentially increase costs. The increased use of alternative data has grown to identify unique trends as well as cut costs. This market is highly unregulated and creates many quality issues – especially since these data sources were previously non-existent or largely underutilised.
Read the whitepaper: Machine Learning & Artificial Intelligence for Trading and Execution
Overcoming the data challenge
Vendors solve the problems related to the overall data architecture in a number of ways. Data quality and normalisation is generally approached by building technologies that convert multiple data sources into a common format. This has always been a vendor requirement for execution management system (EMS) / order management system (OMS) providers as they must interact with different vendors to offer their service. There’s little motivation from markets and data providers to agree on a fully normalised format for their data – because there’s a direct benefit to limiting ease of integration.
Execution (EMS) vendors, however, are at the forefront of consuming a range of data sources to power their trading systems. This is often more varied than the focus that any one client may have on consuming data. Whereas, for an EMS / OMS, each client will have a range of data sources across different asset classes and geographies – all of which must be handled in a common format.
Building a new solution to the problem already faced daily by EMS / OMS providers, brings little value and significant pain. The trading technology provider has become the critical partner that’s able to present a common data interface for a wider range of applications.
A holistic data solution
As volumes and the sources of data continue to increase, this will add even greater pressure to the already limited budgets and resources of banks, brokers, traders, data scientists, chief data officers, quant developers and others – for whom the importance of different types of data cannot be underestimated.
"Data is the foundation of any AI / ML models. These models require a wide source of data including real-time data, historical data and alternative data. AI/ML allows firms to consume and process a huge amount of data to reach actionable decisions."
AI / ML models in trading financial securities depend on good quality data from multiple sources – as well as secure, reliable connectivity to those data sources. In summary, AI / ML needs:
- datasets for learning and testing
- access data for production environments
- connectivity from customer site to the AI / ML trading systems.
Quod Financial and BT Radianz provide a solution to tackling these data challenges quickly and cost effectively. We can give you immediate access to normalised, cleansed data, as well as tooling and storage to minimise costs and improve trading performance.
Quod Order Management System: multi-asset trading and automation
How it works
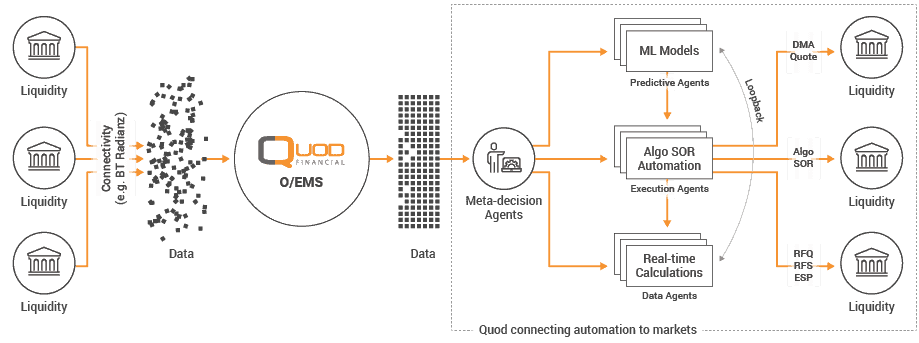
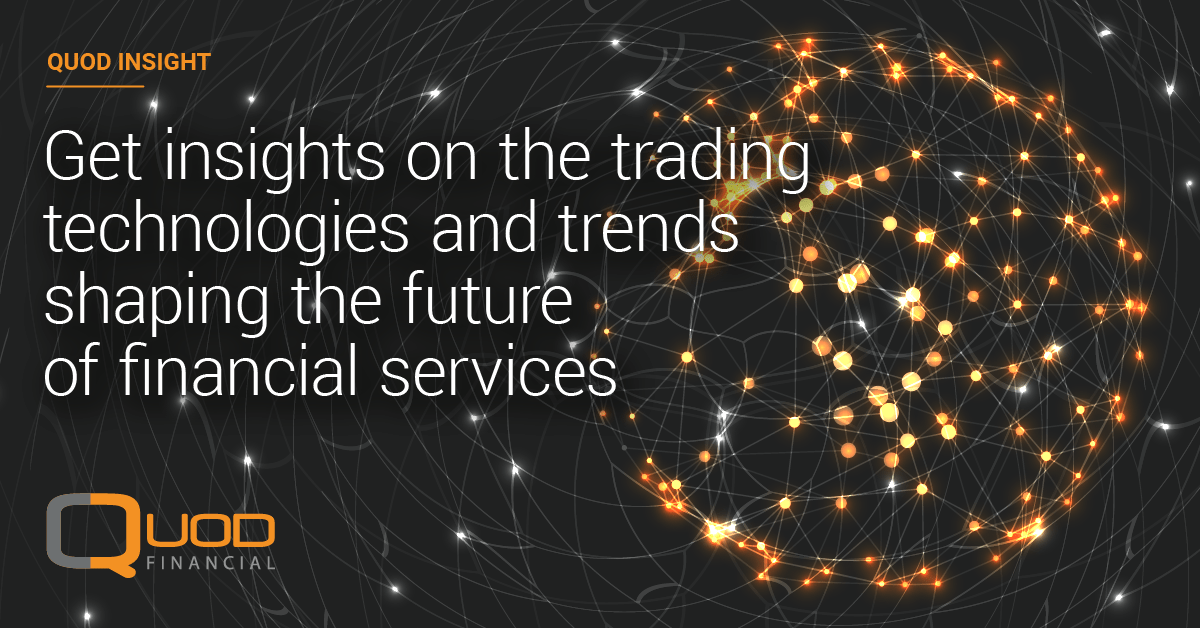